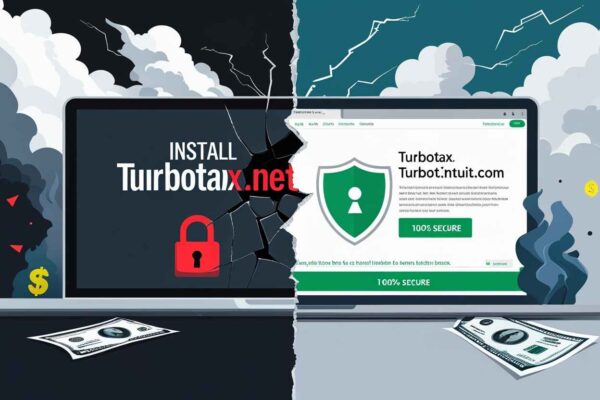
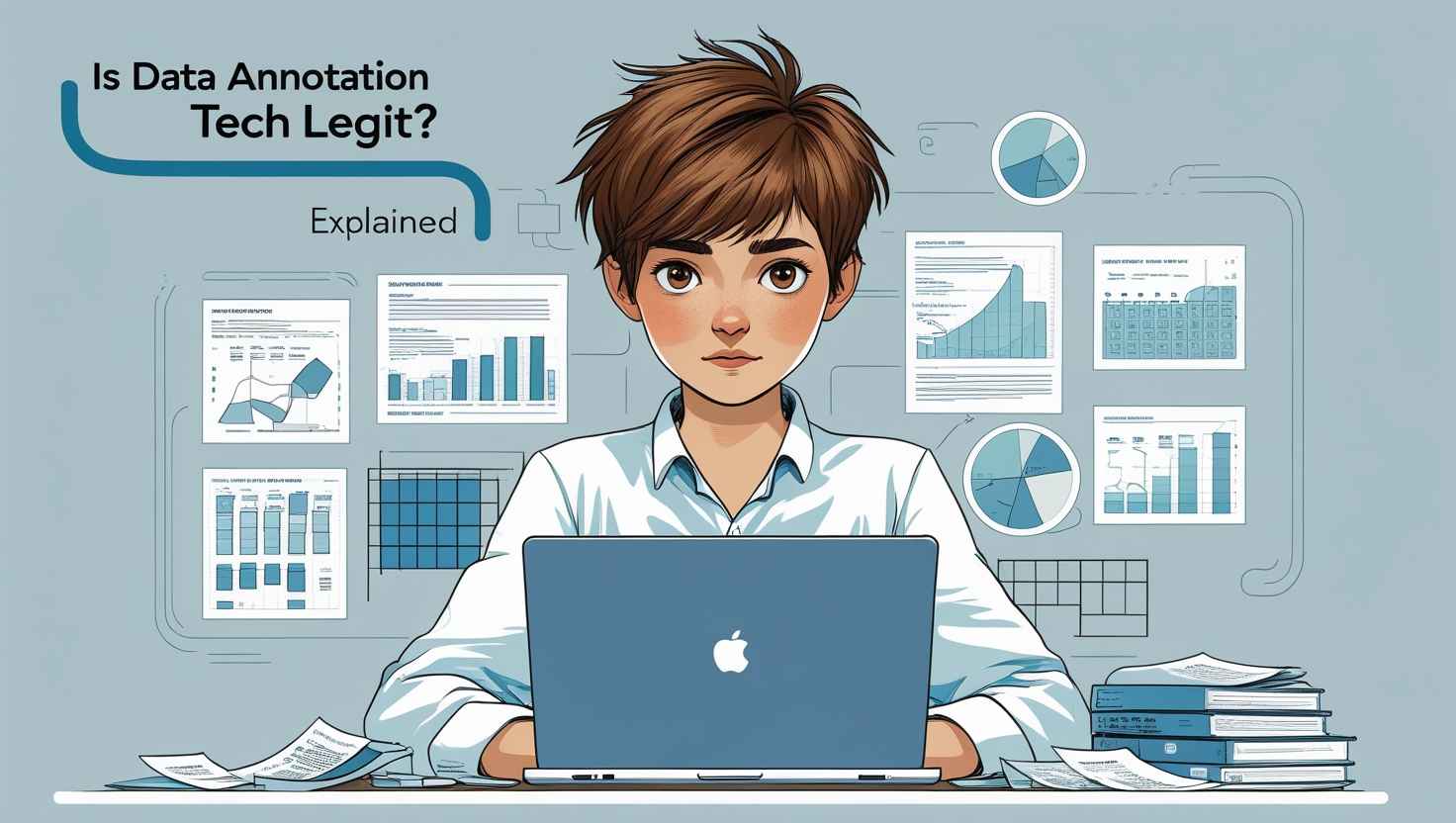
Is Data Annotation Tech Legit? Explained
Introduction
The process of annotating data supports the effective operation of artificial intelligence (AI) and machine learning (ML). The process organizes information for AI models to achieve successful training. The majority of businesses utilize technological data annotation to boost their automated processes and decision systems. The legitimacy of data annotation technology frequently sparks inquiries among people. This article investigates Is Data Annotation Tech Legit? Data annotation from the perspective of its meaning to the industry through explaining typical fraudulent techniques and platform evaluation methods which deliver reputable data annotation services.
Understanding Data Annotation Technology
What is Data Annotation?
Neural programs need labeled raw data to understand information through data annotation processes. Navigation of supervised learning strongly depends on human-labeled data because AI models need this input to identify patterns. The following primary forms make up data annotation:
- Text Annotation: NLP benefits from text annotation through which analysts provide sentiment analysis and named entity recognition and language translation model processing.
- Image Annotation: Artificial intelligence tasks depend on visual marking of image objects to operate computer vision functions including facial recognition and autonomous vehicle navigation and manufacturing defect identification.
- Audio Annotation: Speech transcription and labeling functions as an improvement method for voice recognition programs and sentiment analysis technology and platforms like chatbots.
- Video Annotation: High-quality AI training requires frame tagging and object tracking in videos for producing motion detection systems and security surveillance and creating virtual reality technologies.
AI system development requires different annotation types to fulfill distinct operational purposes. The lack of proper annotation interferes with AI models that need patterns to identify things and develop correct predictions.
Applications Across Industries
Various industries achieve advantages from data annotation processes because the global adoption of AI continues to grow. Some key industries include:
- Healthcare: AI diagnostics and medical imaging services as well as patient monitoring systems require precise data labeling for their operations. The training of disease detection AI models through AI models requires radiologists to annotate X-rays and MRI scans.
- Automotive: Autonomous vehicles depend on top-quality image and video annotations which help them see and identify objects and pedestrians and road signs. Amazon and Waymo use millions of labeled images to develop self-driving technology.
- Finance: Financial institutions alongside banks apply data annotation for creating fraud detection systems while also automating trading processes and enhancing risk evaluation capabilities.
- Retail: Data annotation produces accurate results that enhance both recommended products for customers and AI-based chatbots as well as inventory tracking systems. Amazon along with eBay apply annotated data to optimize their customer service quality.
- Security & Surveillance: Security cameras with AI functions depend on annotated content to find abnormal movements and understand facial features to block entry to unapproved personnel.
Data annotation plays a vital role in everyday AI applications because their growing popularity shows the clear demand across many industrial sectors.
Role in AI Development
An effective operation of AI models depends on high-quality datasets with proper annotations. The data annotation efforts of technology giants Microsoft and Google along with Amazon generate important upgrades in their products that use artificial intelligence. AI models fail to produce accurate predictions when they cannot receive precise labels for their information generalization process.
AI models demand ongoing updates with fresh data for their relevant operation. Both Alexa and Siri obtain updates from newly labeled speech data to better understand different accents and slang within their training regime.
Is Data Annotation Tech Legit?
Evidence Supporting Legitimacy
The data annotation industry continues to expand at a quick rate across the worldwide marketplace. Market research indicates that industry value will exceed billions because of growing AI adoption. The data annotation industry attracts investments from several businesses.
- Amazon Mechanical Turk: Crowdsourcing for AI training data.
- Scale AI: Scale AI serves as a source for autonomous systems which delivers high-quality labeled datasets.
- Appen: It provides NLP and computer vision training data solutions to customers through their AI data services department.
- Lionbridge AI: Supporting AI training with multilingual data annotation services.
- Clickworker: It serves as a crowd-based platform which delivers data labeling services at an expandable scale.
Through their operations these organizations maintain the valid position of data annotation within the industry. Data annotation technology gains credibility through companies that focus on quality control, ethical labor operations and transparent data handling practices.
Common Misconceptions and Scams
Data annotation remains a legitimate industry although certain fraudulent activities exist which target workers who lack discrimination. Be cautious of:
- Fake job listings: High-paying data annotation positions are deceptive job offers since the companies withhold payments to their workers. The hiring process involves use of social media platforms and job search boards to find new candidates.
- Unrealistic earnings claims: Some cybercriminals attempt to inflate the potential income levels found in data annotation work opportunities. A legitimate platform will provide fair pay but no business organization can assure substantial profits through minimal work.
- Upfront fees: To obtain access to job opportunities you must not provide payment to any legitimate working platform. Steer clear from any company which requires payment of membership fees or makes demands for deposits.
- Personal information requests: Stake sensitive information from platforms which you do not recognize. The scammers attempt to obtain your passport information along with bank details and ID documentation when pretending to confirm your identity.
Research the legitimacy of any company before joining to prevent scammers.
Also Read: Izonemedia360.com Tech: Innovation and Digital Solutions
Is Data Annotation Tech Legit? Evaluating Legitimate Platforms
Criteria for Trustworthy Platforms
The search for trustworthy data annotation platforms requires you to check the following factors:
- Company Reputation: Reviewers on Glassdoor Trustpilot and Reddit discuss company practices with existing workers while you can find reliable information there.
- Payment Transparency: All users should understand the payment process method as well as the timing of payment transfers. Payment policies together with payment methods should be presented in a straightforward manner by platforms.
- User Feedback: The experiences of other workers who use data annotation platforms are best learned through participation in related communities and forums.
- Terms & Conditions: A genuine platform establishes direct rules for work conduct which include data privacy regulations.
Case Study: DataAnnotation.tech
DataAnnotation.tech represents a website which emphasizes quality control as its main focus. The platform integrates accurate product labeling and protects worker privacy and gives them proper remuneration. Users need to understand how the operation works to gauge its validity and authenticity.
Ethical Considerations in Choosing Providers
The data annotation business cannot overlook multiple ethical issues that persist in its operations. Selecting your provider requires several essential points to take into account.
- Fair wages: Your organization should pay workers appropriate compensation for the work they perform.
- Good working conditions: Identify platforms which demonstrate genuine respect for their precious team members.
- Data privacy: Each company must protect personal data and keep to established legal requirements.
- Avoiding exploitation: Check that annotation jobs outsourcing companies maintain ethical labor practices because many annotation jobs are sent outside the company and primarily located in other nations.
Challenges Facing the Industry
Quality Control Challenges
Ensuring Consistency
The different annotators who work on data labeling produce inconsistent results because they use varying methods to label things. Quality maintenance in companies happens through the combination of multiple reviewers along with AI-assisted annotation tools.
Addressing Bias Issues
Bias present within the data produces negative consequences for decisions made using AI systems. Organizations that want to prevent faulty model development alongside discriminatory outcomes must maintain diverse datasets. The training of facial recognition models using limited ethnic populations has produced discriminatory patterns during operation. Team annotation diversity establishes a solution to combat these types of problems.
Ethical Concerns
Labor Practices
Various data annotation work gets sent to less developed nations through outsourcing contracts. The payment structure and working environment of employees should be maintained at fair levels with ethical integrity. The ethical issues surrounding discrimination rise when companies provide minimum wage and force workers to extend their shifts beyond reasonable limits.
Privacy Issues
Data containing sensitive information gets regularly used for annotation purposes. To keep information secure and private companies need robust data protection policies that must be deployed. Anymployee who handles medical data and financial data needs to maintain absolute confidentiality according to company standards.
Technological Advancements Impacting Quality Control
The integration of AI tools for annotation purposes produces enhanced quality together with better operational speeds. The process requires human supervision to guarantee highest possible standards of labeled data quality. Automation streamlines the labeling procedures yet it fails to deliver the sophisticated understanding which human annotators have.
Future Trends in Data Annotation Technology
Automation & Hybrid Models
The upcoming data annotation systems will integrate automation systems. AI annotation tools accelerate the process and still require human workers at critical points such as complex task evaluation and quality control.
Growth & Demand
Businesses in healthcare and automotive sectors alongside finance will sustain their demand for high-quality annotated data because of growing AI adoption. Businesses need quality solutions that combine price efficiency with the ability to scale in order to fulfill upcoming data demands.
Emerging Technologies
New innovative annotation tools and technological advancements will lead to improved data annotation efficiency because
- AI-assisted annotation: Artificial intelligence models help human annotators who work in data labeling operations.
- Blockchain for data integrity: Ensuring secure and tamper-proof annotations.
- Advanced labeling techniques: Semi-supervised learning methods help decrease the requirements for human-based annotation work.
Conclusion
Is data annotation tech legit? Yes, the development of artificial intelligence depends on data annotation which provides high-quality labeled data for various industries. Data annotation serves as a legitimate industry yet it contains both ethical and fraudulent activities. Businesses and individuals who perform research along with platform credibility checks and ethical understanding can participate in safe data annotation work which supports AI-driven solutions of tomorrow.
Also Read: Tech theboringmagazine: A Deep Dive into Tech’s Home
FAQs About Is Data Annotation Tech Legit?
A: Data annotation involves labeling datasets so AI systems can understand them better.
A: Yes, it’s a crucial step in AI development used by reputable companies worldwide.
A: Text, images, audio, and video data all need annotations for various AI applications.
A: Annotated datasets provide context for machines to learn from real-world scenarios effectively.
A: Healthcare, automotive, finance sectors benefit significantly from annotated datasets.
A: Yes, some unverified platforms offer fake jobs or non-payment schemes; research thoroughly before engaging with any company.
A: Check reviews on Glassdoor or Trustpilot and ensure clear payment policies are in place.
A: Automation will enhance efficiency but won’t replace human oversight entirely; hybrid models combining both will prevail.
A: Ensure fair labor practices and privacy protection measures are implemented by the provider.
A: Expect more hybrid models integrating automation with human judgment for improved accuracy and efficiency.